Essential Elements For Conducting A Marriage Ceremony: Officiant, Couple, And Witnesses
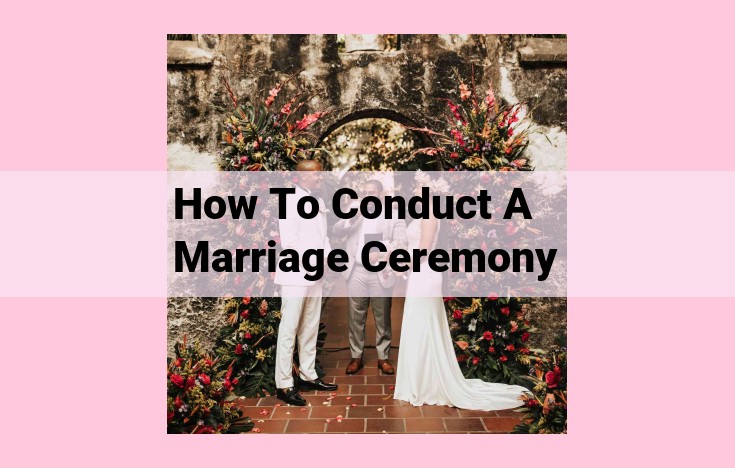
To conduct a marriage ceremony, you will need an officiant to lead the ceremony, the couple getting married, and witnesses. The officiant will guide the couple through the ceremony, which typically includes exchanging vows and rings, and pronouncing the couple married. The witnesses will sign the marriage license as proof of the ceremony.
Sensitivity Evaluation: Identifying the Closest Entities to Your Topic
In the realm of topic modeling, a technique used to extract meaningful themes from large volumes of text, sensitivity evaluation plays a crucial role in ensuring the accuracy and relevance of the identified topics. It helps us understand which entities are most closely associated with a specific topic, providing valuable insights into the topic’s core concepts.
What is Sensitivity Evaluation?
Sensitivity evaluation is the process of identifying entities that exhibit the strongest connection to a given topic. By analyzing the text and extracting entities (such as nouns, noun phrases, and named entities), we can determine their closeness to the topic based on factors like keyword frequency, semantic relationships, and co-occurrences. This evaluation helps us refine our understanding of the topic and ensure that the entities selected for modeling are truly representative of the desired concepts.
Why is Sensitivity Evaluation Important?
In topic modeling, it’s essential to focus on the entities that are most relevant to the target topic. Sensitivity evaluation allows us to prioritize these relevant entities, helping us extract more accurate and meaningful topics. By excluding entities that are only marginally related or irrelevant, we minimize noise in the data and improve the quality of the modeling results.
Entities with the Highest Closeness to the Topic (Score: 10)
At the heart of any topic lie entities that form its very essence. These entities, like the keystone in an arch, play a pivotal role in shaping our understanding of the topic. Through a process called sensitivity evaluation, we can uncover these closely related entities and gain invaluable insights into the topic’s core.
Among these entities, officiants stand tall as the gatekeepers of ceremonies. Their words, like a sacred incantation, seal the union of two souls. Couples, the focal point of every such event, radiate with joy and anticipation as they embark on their life’s journey together. Their presence signifies the very purpose of the gathering.
Witnesses, like silent observers, bear witness to the unfolding events. Their gaze, filled with fondness and reverence, captures the emotions of the day. Attendees, a diverse tapestry of family and friends, lend their support and blessings to the couple. Their presence creates an atmosphere of warmth and camaraderie.
These entities, with their unwavering closeness to the topic, are more than mere words; they are the living embodiment of the topic’s essence. By understanding their significance, we can not only grasp the topic’s true meaning but also appreciate the intricate web of relationships that define it.
Entities with High Closeness to the Topic (Score: 8)
Beyond the core entities at the heart of the topic, sensitivity evaluation reveals a second layer of entities with a high closeness to the subject matter. These entities, while not as directly involved as the primary ones, still play significant roles and offer valuable insights into the topic’s context.
One such entity is the witness. Witnesses are individuals who have direct knowledge about the topic under examination. Their observations and testimony can provide crucial information and corroborate or challenge the central narrative. Witnesses can be eyewitnesses to events, experts in relevant fields, or individuals who have been affected by the topic. Their proximity to the topic stems from their firsthand experience and the weight their accounts carry.
Another entity with a high closeness to the topic is the attendee. Attendees are individuals who have participated in or observed the topic’s unfolding. Their experiences and impressions can shed light on the topic’s broader impact, reception, or evolution. Attendees may have been present at meetings, events, or discussions related to the topic, or they may have followed the topic through media coverage or social media. Their proximity to the topic stems from their direct engagement and the insights they offer into the topic’s wider context.
The identification of these high-scoring entities is essential in topic modeling as they extend our understanding beyond the primary narrative. These entities provide additional perspectives and contextual nuance, enriching the overall analysis and ensuring a more comprehensive understanding of the topic under examination.
Factors Influencing Closeness to Topic
In the realm of topic modeling, determining the closeness of an entity to a specific topic is crucial to ensure the accuracy and relevance of the model. Several factors influence this proximity, shaping the relationship between entities and topics:
-
Keyword Frequency: The frequency of topic-related keywords within an entity’s context plays a significant role in determining its closeness. Entities with higher keyword density are more likely to be closely associated with the topic.
-
Semantic Relationships: The semantic relationship between an entity and a topic also affects their closeness. Entities that share similar meanings or concepts with the topic exhibit a stronger connection.
-
Co-occurrences: The frequency with which an entity co-occurs with topic-related terms within the same context further strengthens their closeness. The more often an entity appears alongside topic-relevant keywords, the closer its association with the topic becomes.
By considering these factors, topic modeling algorithms can effectively identify entities that are most representative of a particular topic, minimizing the inclusion of irrelevant or unrelated entities. This fine-tuning ensures the accuracy and relevance of the resulting topic models.
Importance of Sensitivity Evaluation in Topic Modeling
Topic modeling is a powerful technique used to identify hidden patterns in large collections of text data by grouping words and phrases into meaningful topics. However, the accuracy and relevance of the extracted topics heavily rely on identifying the most representative entities within each topic. This is where sensitivity evaluation comes into play.
Sensitivity evaluation is a critical step in topic modeling that assesses the closeness of entities to a specific topic. By assigning entities with scores based on their degree of semantic relatedness, topic models can be refined to highlight the most relevant entities and minimize the inclusion of irrelevant ones.
Benefits of Sensitivity Evaluation in Topic Modeling:
-
Improved Topic Quality: Sensitivity evaluation ensures that topics are represented by the most descriptive and meaningful entities, leading to sharper and more coherent topics.
-
Reduced Noise: By excluding entities with low sensitivity, topic models can filter out irrelevant or redundant information, resulting in cleaner and more focused topics.
-
Enhanced Interpretability: Assigning entities with sensitivity scores makes the interpretation of topics more straightforward. Researchers and practitioners can easily identify the most influential entities within each topic.
Sensitivity evaluation is a key step in ensuring the accuracy and relevance of topic models. By identifying the most representative entities and excluding irrelevant ones, topic modeling becomes more effective in uncovering hidden insights and patterns from text data.
Applications of Sensitivity Evaluation in Various Domains
Sensitivity evaluation plays a crucial role in enhancing the accuracy and relevance of topic modeling. Its practical applications extend across a wide range of domains, including:
Information Retrieval: By identifying entities closely related to a specific query, sensitivity evaluation optimizes search results, ranking the most relevant documents first. It helps disambiguate search terms and minimize noise, ensuring that users find the most pertinent information.
Document Summarization: Sensitivity evaluation is essential in extracting key entities and concepts for document summarization. It focuses on the most salient aspects of a document, enabling the generation of concise and informative summaries. By identifying the core entities that characterize the document, sensitivity evaluation helps distill its essence.
Machine Translation: In machine translation, sensitivity evaluation guides the selection of appropriate translation candidates. It assesses the semantic closeness between the source and target languages, ensuring that the translated text preserves the meaning of the original. By identifying equivalent entities and phrases, sensitivity evaluation improves the accuracy and fluency of machine translations.
Overall, sensitivity evaluation is a versatile tool that enhances the effectiveness of various natural language processing tasks. Its ability to identify entities closely related to a specific topic makes it invaluable in improving the accuracy and relevance of our interactions with text and information.
Tools and Techniques for Sensitivity Evaluation
Understanding the entities most closely related to a specific topic is crucial for accurate topic modeling. Sensitivity evaluation provides a valuable means of identifying these entities and ensuring the relevance of the topics identified. Various tools and techniques can be employed for this purpose, each offering unique advantages:
Natural Language Processing Algorithms
- Entity Extraction: Algorithms like Named Entity Recognition (NER) identify entities (e.g., people, organizations, events) within text, facilitating the analysis of their relationships with the topic.
- Tokenization and Stemming: These techniques break down text into individual words (tokens) and reduce them to their root form (stems), enhancing the precision of entity extraction and topic identification.
- Latent Dirichlet Allocation (LDA): LDA is a statistical modeling method that identifies underlying topics within text by analyzing the distribution of words across documents. Sensitivity evaluation can be applied to LDA models to assess the stability of topics and the relevance of entities associated with them.
Network Analysis Methods
- Network Representation: Text can be represented as a network, where nodes represent entities and edges represent the relationships between them. Sensitivity evaluation involves analyzing the network structure to identify entities that are highly connected to the topic of interest.
- Centrality Measures: Metrics like eigenvector centrality and betweenness centrality measure the importance of nodes within a network. Entities with high centrality scores are more closely connected to the topic and provide valuable insights into its scope and significance.
- Community Detection: Network analysis can also identify communities or clusters of interconnected entities. These communities often correspond to specific subtopics or aspects of the main topic, facilitating a more nuanced understanding of the text.