Dishwasher Life Expectancy: How Long Do They Last?
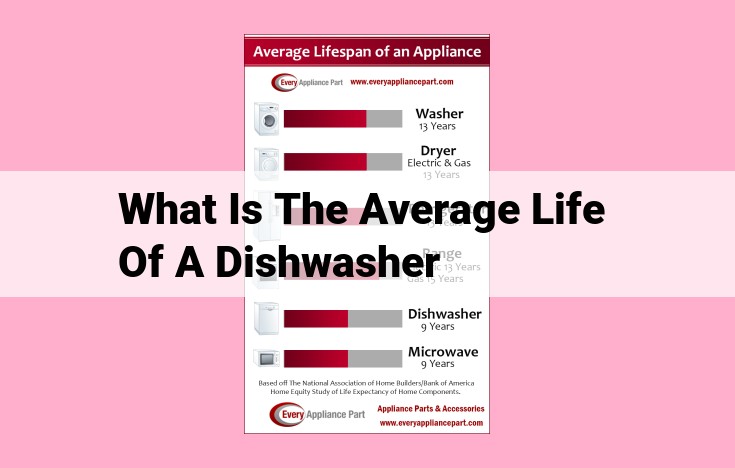
The average life of a dishwasher varies depending on factors such as brand and usage. According to the Association of Home Appliance Manufacturers (AHAM), the average life expectancy of a dishwasher is between 8 and 10 years. Higher-end models with advanced features may have a longer life expectancy, while budget-friendly options may have a shorter lifespan. Regular maintenance and proper use can prolong the lifespan of a dishwasher, extending its usefulness and saving you replacement costs.
Unraveling Table Relationships: A Guide to Building Contextual Connections
When working with data, understanding the relationships between different entities is crucial for accurate analysis and meaningful insights. Table relationships provide a structured way to define and explore these connections, creating a solid foundation for data-driven decision-making.
This blog post provides an outline for establishing table relationships within a specific context. We will delve into the key concepts and components of table relationships, with a focus on identifying primary entities, secondary entities, and relationship strength. By understanding these foundational elements, you can effectively establish relationships between entities, unlocking the power of your data.
Understanding Primary Entities in Table Relationships
In the realm of data analysis, table relationships play a pivotal role in organizing and interpreting data effectively. These relationships serve as a roadmap, guiding analysts through the connections between different entities within a specific context.
One of the fundamental components of table relationships is the concept of primary entities. These are entities that stand at the heart of the relationship table, representing the main subjects or actors whose interactions we seek to understand.
In our illustrative example, we embark on a quest to unveil the relationships between home appliance manufacturers. Our primary entities are renowned brands like KitchenAid, Bosch, LG, and Whirlpool. These industry titans occupy the center stage of our analysis, as we delve into their interconnectedness.
To ensure a meaningful and insightful exploration, we impose a closeness rating requirement. This rating serves as a threshold, guiding us toward relationships that exhibit a specified level of significance. In our case, we stipulate that only relationships with a closeness rating of 8 or higher will be deemed worthy of consideration.
By focusing on primary entities and applying a closeness rating filter, we narrow our scope to the most relevant and substantial relationships. This enables us to distill the vast expanse of data into a manageable and illuminating subset that can empower our analysis and uncover valuable insights.
Unveiling the Essence of Secondary Entities in Table Relationships
In the fascinating realm of data analysis, table relationships hold immense significance, enabling us to unravel the intricate connections between different entities. These relationships play a pivotal role in organizing and interpreting data, offering valuable insights that would otherwise remain hidden from our grasp.
To establish these relationships, we must first identify the primary entities – the central figures around which the data revolves. In our example, we focus on the world of home appliances, with KitchenAid, Bosch, LG, and Whirlpool taking center stage as our primary entities.
But the story doesn’t end there. Often, there are other secondary entities that play a supporting role in these relationships. These entities provide additional context and contribute to the overall understanding of the data. In our case, the Association of Home Appliance Manufacturers (AHAM) emerges as our secondary entity.
AHAM serves as an umbrella organization representing the interests of home appliance manufacturers. Its presence in the relationship table enriches our analysis by providing a collective perspective on industry trends, regulations, and consumer preferences. By including AHAM, we gain a deeper understanding of the factors that influence the primary entities and their interactions.
The closeness rating serves as a measure of the strength of the relationship between entities. In our example, we stipulate that the closeness rating for AHAM should be 8. This threshold indicates a strong connection between the secondary entity and the primary entities, suggesting that AHAM exerts a significant influence on the home appliance industry.
Understanding the role of secondary entities is fundamental in data analysis. They provide additional layers of information, enriching our comprehension of the data and enabling us to make more informed decisions. By considering both primary and secondary entities, we gain a holistic view of the relationships within our dataset, unlocking a wealth of valuable insights.
Relationship Strength: Measuring the Bond Between Entities
In the world of data analysis, understanding the relationships between entities is crucial. Closeness rating emerges as a valuable metric to quantify the strength of these relationships, providing insights into the interconnectedness of different data points.
Closeness rating is a numerical value assigned to a relationship, indicating the level of association between the entities involved. Higher closeness ratings represent stronger relationships, while lower ratings indicate weaker ones. For instance, a closeness rating of 9 signifies a very strong connection, while a rating of 4 suggests a relatively weak relationship.
In our example, we consider the relationship between kitchen appliance manufacturers and the Association of Home Appliance Manufacturers (AHAM). The closeness rating for this relationship is set at 8, indicating a strong association between the two entities. This implies that the manufacturers are actively engaged with AHAM, participating in its activities and adhering to its standards.
Understanding relationship strength enables data analysts to identify and prioritize the most significant connections within their datasets. By filtering and sorting relationships based on closeness ratings, they can focus on the most impactful ones for their analysis. This approach helps in uncovering patterns, trends, and insights that would otherwise remain hidden.